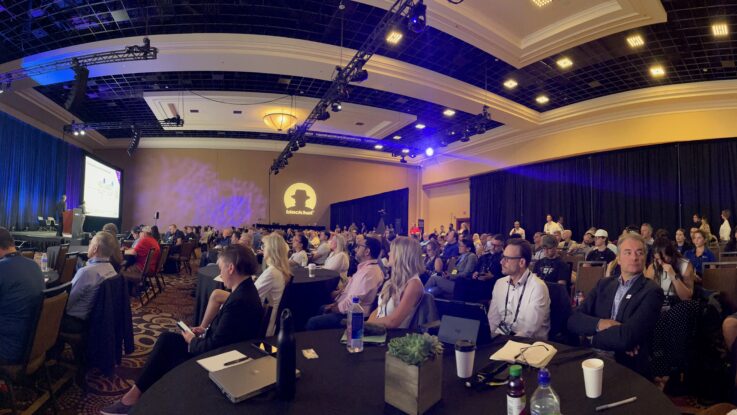
It’s been a rapid evolution, even for the IT industry. At 2022’s edition of Black Hat, CISOs were saying that they didn’t want to hear the letters “AI”; at RSAC 2023, practically everyone was talking about generative AI and speculating on the huge changes it would mark for the security industry; at Black Hat USA 2023, there was still talk about generative AI, but with conversations that centered on managing the technology as an aid to human operators and working within the limits of AI engines. It shows, overall, a very quick turn from breathless hype to more useful realism.
The realism is welcomed because generative AI is absolutely going to be a feature of cybersecurity products, services, and operations in the coming years. Among the reasons that is true is the reality that a shortage of cybersecurity professionals will also be a feature of the industry for years to come. With generative AI use focused on amplifying the effectiveness of cybersecurity professionals, rather than replacing FTEs (full-time equivalents or full-time employees), I heard no one discussing easing the talent shortage by replacing humans with generative AI. What I heard a great deal of was using generative AI to make each cybersecurity professional more effective — especially in making Tier 1 analysts as effective as “Tier 1.5 analysts,” as these less-experienced analysts are able to provide more context, more certainty, and more prescriptive options to higher-tier analysts as they move alerts up the chain
Gotta Know the Limitations
Part of the conversation around how generative AI will be used was an acknowledgment of the limitations of the technology. These weren’t “we’ll probably escape the future shown in The Matrix” discussions, they were frank conversations about the capabilities and uses that are legitimate goals for enterprises deploying the technology.
Two of the limitations I heard discussed bear talking about here. One has to do with how the models are trained, while the other focuses on how humans respond to the technology. On the first issue, there was great agreement that no AI deployment can be better than the data on which it is trained. Alongside that was the recognition that the push for larger data sets can run head-on into concerns about privacy, data security, and intellectual property protection. I’m hearing more and more companies talk about “domain expertise” in conjunction with generative AI: limiting the scope of an AI instance to a single topic or area of interest and making sure it is optimally trained for prompts on that subject. Expect to hear much more on this in coming months.
The second limitation is called the “black box” limitation. Put simply, people tend not to trust magic, and AI engines are the deepest sort of magic for most executives and employees. In order to foster trust in the results from AI, security and IT departments alike will need to expand the transparency around how the models are trained, generated, and used. Remember that generative AI is going to be used primarily as an aid to human workers. If those workers don’t trust the responses they get from prompts, that aid will be incredibly limited.
Define Your Terms
There was one point on which confusion was still in evidence at both conferences: What did someone mean when they said “AI”? In most cases, people were talking about generative (or large language model aka LLM) AI when discussing the possibilities of the technology, even if they simply said “AI”. Others, hearing the two simple letters, would point out that AI had been part of their product or service for years. The disconnect highlighted the fact that it’s going to be critical to define terms or be very specific when talking about AI for some time to come.
For example, the AI that has been used in security products for years uses much smaller models than generative AI, tends to generate responses much faster, and is quite useful for automation. Put another way, it’s useful for very quickly finding the answer to a very specific question asked over and over again. Generative AI, on the other hand, can respond to a broader set of questions using a model built from huge data sets. It does not, however, tend to consistently generate the response quickly enough to make it a superb tool for automation.
There were many more conversations, and there will be many more articles, but LLM AI is here to stay as a topic in cybersecurity. Get ready for the conversations to come.