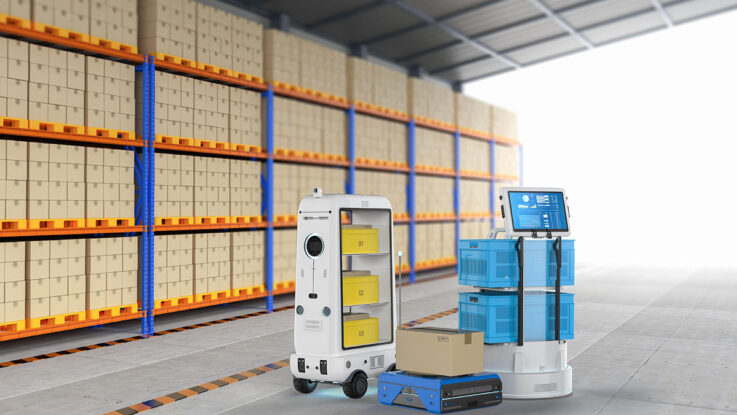
There is no doubt that generative artificial intelligence (GenAI) is going to change how business gets done. Research firms are estimating huge productivity gains across all sectors that, if fulfilled, would completely transform every industry. With such great potential gain, it is clear why every enterprise is striving to enable their teams to build AI-powered applications as fast as possible. However, security teams must act now to ensure these apps will hold up to scrutiny.
The Race to Capture AI Business Value First
Some enterprises have already built hundreds of AI-powered apps so far. The rate of development is just incredible, with notable examples like Microsoft releasing Copilot applications at a rate far beyond what a huge enterprise typically delivers.
Because of the immaturity of the frameworks and tooling around AI app development, these are being built with a wide range of technologies. Development frameworks that build on top of the few fundamental models are numerous and vary significantly, and they keep on popping up. Frameworks such as LangChain and AutoGPT have gained significant popularity at an unprecedented pace. In a major enterprise, you can easily expect to find tens of different frameworks being used to build these applications.
The first organizations that are able to capture productivity gains from AI before others will have a huge win. Therefore, we are taking part in a race where we have to make do with the frameworks available right now and just get things done. It will probably take a long time for frameworks to standardize, and by that time you’ll already be late to the game.
We have to face reality: Business is being reimagined — with unproven tools, frameworks, and threat models — at an unprecedented pace.
Security: Where Do We Even Begin?
Building so many new applications in such a short time frame has huge security implications. First, these are just more applications, with the same security risks as any other application introduces; they need to get identity, dataflow, and secret management right, to name a few concerns. Second, GenAI creates some unique security challenges, which frameworks such as the OWASP LLM Top 10 help to capture and educate on.
Advanced security organizations, in collaboration with IT, are putting together dedicated centers to inventory, assess, and secure these applications. Note that these require creating entirely new processes and newly delegated responsibilities. Ideally, these centers can act as an enabling resource for developers, offering threat modeling and design review services to ensure secure standards are met.
Creating a centralized resource is not an easy feat. Finding all AI-powered projects across an enterprise is a huge challenge, as inventory always is. Developing the technical skills required to audit these applications is difficult as well — especially due to the proliferation of different AI frameworks, each with its own quirks and gotchas. Monitoring these apps in production is yet another challenge, both from a technical perspective of getting the right data from immature development frameworks and from the security analysis perspective of knowing what to look for.
These are not insurmountable challenges, however. In fact, they follow the typical application security problem formula of inventory, security assessment, and runtime protection. To get ahead and enable our business to capture the AI revolution first, we have to start making headway in solving those problems.