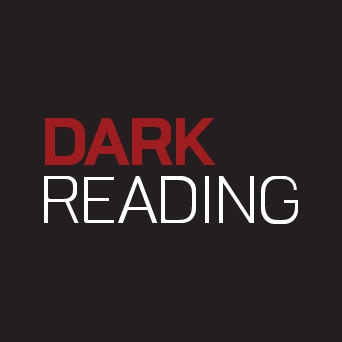
In their 1955 proposal for a summer research project on artificial intelligence (AI), researchers at Dartmouth Conference predicted “…every aspect of learning or any other feature of intelligence can in principle be so precisely described that a machine can be made to simulate it.” In the decades that followed, AI research continued at what seemed a glacial pace, always promising a breakthrough in the near future — until language tools like ChatGPT finally exploded on the scene.
As we find our footing with AI today, it’s clear that we’re facing risks as well as benefits. A recent survey of 1,500 IT professionals showed that nearly half (49%) of decision-makers are concerned the new tools will help cybercriminals, but a full 82% said they plan to integrate AI into their security programs over the next two years.
AI Is the Hero to Embrace, Not the Villain to Defeat
Rapid advancements in AI since the 1950s set the stage for growth — with no signs of slowing. The global market for AI-based security tools is expected to reach $133 billion by 2030 with more integration and use in daily DevSecOps workflows. As the industry incorporates AI and machine learning (ML) into more processes, we’re seeing more and more opportunities to engage AI as a force for good, including the following.
Faster and More Accurate Configuration for Security Tools
To be effective, most security technologies today require a lot of manual fine-tuning, often through sophisticated parameter tweaks. Depending on the tool, these can affect what incidents are reported, what vulnerabilities a tool finds, or how issue priorities are determined. All these manual tweaks are time-consuming and can leave you exposed to threats until the right configurations are in place.
That’s where machine learning comes to the rescue. In this case, ML can continually optimize parameters, for instance by prioritizing items in a scanning queue to ensure operations run as efficiently as possible. Once they automate these configuration tasks, future cybersecurity teams will spend far less time on tedious manual work.
Improved Risk Scoring and Threat Intelligence
Modern scanning tools typically provide a risk assessment once a scan is complete. This shows the various levels of security protection and potential risk across your applications, websites, and networks for a better view of your threat exposure. Different tools will factor in different data, such as the technical severity of vulnerabilities, their potential exploitability, their impact on business if exploited, and their importance for your overall security posture.
While useful, these assessments don’t always provide deeper context or guidance, which are necessary for security to keep up with fast-paced software development. In the coming years, security tools will extensively use machine learning for evaluating risk and managing threats. As machine-generated results continue to improve in scope and quality, they will support more accurate, data-based decision making by showing which issues are actionable and in what order they should be addressed to minimize risk.
Putting a Sharper Edge on Security Testing
As ChatGPT and similar tools powered by large language models (LLMs) are refined and continue to gain popularity, we can expect easier access to ever more accurate insights. By encouraging engineers and developers to safely use AI and ML in their work, these machine-driven solutions should also provide better and more useful insights over time — even more so when accurate security guidance is included in the mix.
When it comes to security testing, training AI/ML tools to become sharper will further help with fine-tuning static application security testing (SAST) and dynamic application security testing (DAST) tools. Ultimately that means increasing control and precision for scan results, providing reliable intelligence into current and future risks, and improving the efficacy of vulnerability hunting.
Fewer False Positives for Less Manual Verification
False positives are a persistent challenge for security and one that frequently translates into hours of manual work checking scan results. As teams spend valuable time verifying reports that should already be as accurate as possible, they can lose confidence in security tools and processes. Fortunately, a recent study by IBM showed that AI can reduce false positives by 65%, freeing resources for activities that add business value.
As the technology progresses, business and operational leaders will have the reliable data they need to confidently make decisions based on accurate AI/ML results. These outputs will harness the power of learning systems to deliver clear and actionable vulnerability reports, allowing DevSecOps teams to focus on what matters most: building and delivering innovative applications.
Winning the Race to AI Supremacy in Security
From threat identification to tool configuration, we’re already seeing tangible impacts of AI in cybersecurity. Where researchers at the Dartmouth Conference nearly 70 years ago were only speculating, cybersecurity professionals today should look for far more tangible opportunities to incorporate AI and ML into their operations and strategies. If we can realize the potential of existing and emerging tools to continuously improve cybersecurity, AI can truly be one of the good guys.
About the Author
Frank Catucci is a global application security technical leader with over 20 years of experience designing scalable application security-specific architecture, partnering with cross-functional engineering and product teams. Frank is a past OWASP chapter president and contributor to the OWASP bug bounty initiative and most recently was the Head of Application & Product Security at Data Robot. Prior to that role, Frank was the Sr. Director of Application Security & DevSecOps and Security Researcher at Gartner, and was also the Director of Application Security for Qualys. Outside of work and hacking things, Frank and his wife maintain a family farm. He is an avid outdoors fan and loves all types of fishing, boating, watersports, hiking, camping, and especially dirt bikes and motorcycles.