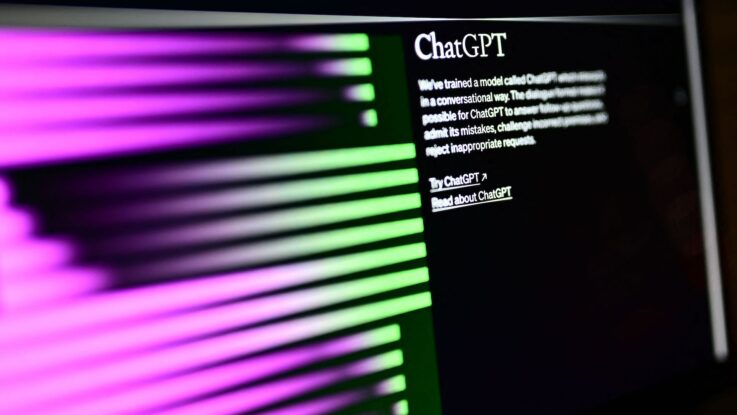
Everywhere you look across the cybersecurity industry — on conference stages, trade show floors or in headlines — the biggest companies in the business are claiming that generative AI is about to change everything you’ve ever known about defending networks and outsmarting hackers.
Whether it’s Microsoft’s Security Copilot, Google’s security-focused large language model, Recorded Future’s AI-assistant for threat intelligence analysts, IBM’s new AI-powered security offering or a fresh machine learning tool from Veracode to spot flaws in code, tech companies are tripping over one another to roll out their latest AI offerings for cybersecurity.
And at last month’s RSA Conference — the who’s-who gathering of cybersecurity pros in San Francisco — you couldn’t walk more than a few feet on the showroom floor without bumping into a salesperson touting their firm’s new AI-enabled product. From sensational advertising, to bombastic pitches to more measured talks from top national security officials, AI was on everyone’s lips.
Recent years’ rapid advances in machine learning have made the potential power of AI blindingly obvious. What’s much less obvious is how that technology is going to be usefully deployed in security contexts and whether it will deliver the major breakthroughs its biggest proponents promise.
Over the course of a dozen interviews, researchers, investors, government officials and cybersecurity executives overwhelmingly say they are eyeing generative AI’s defensive potential with a mix of skepticism and excitement. Their skepticism is rooted in a suspicion that the marketing hype is misrepresenting what the technology can actually do and a sense that AI may even introduce a new set of poorly understood security vulnerabilities.
But that skepticism is tempered by real excitement. By processing human language as it is actually spoken, rather than in code, natural language processing techniques may enable humans and machines to interact in new ways with unpredictable benefits. “This is one of those moments where we see a fundamental shift in human computer interaction, where the computer is more amenable to the way that we naturally do things,” said Juan Andres Guerrero-Saade, the senior director of SentinelLabs, the research division of the cybersecurity firm SentinelOne.
For veterans of the cybersecurity industry, the intense hype around AI can feel like deja vu. Recent advances in generative AI — tools that can replicate human speech and interact with the user — have captured public attention, but the machine learning technologies that underpin it have been widely deployed by cybersecurity firms in the past decade. Machine learning tools already power anti-virus, spam-filtering and phishing-detection tools, and the notion of “intelligent” cyberdefense — a defense that uses machine learning to adapt to attack patterns — has become a marketing staple of the cybersecurity industry.
“These machine learning tools are fantastic at saying here’s a pattern that no human is going to have been able to find in all of this massive data,” says Diana Kelley, the chief information security officer at Protect AI, a cybersecurity company.
In cybersecurity contexts, machine learning tools have sat mostly in the back office, powering essential functions, but the revolution in generative AI may change that. This is largely due to the aggressiveness with which the industry’s leader, OpenAI, has released its generative AI products.
As the technology has advanced in recent years, AI incumbents such as Google, which pioneered many of the technical advances that make possible today’s generative AI tools, have hesitated to release their products into the wild. OpenAI, by contrast, has made its AI tools far more readily available and built slick user interfaces that make working with their language models incredibly easy. Microsoft has poured billions of dollars of investments and cloud computing resources into OpenAI’s work and is now integrating the start-up’s large language models into its product offerings, giving OpenAI access to a massive customer base.
That’s left competitors playing catch-up. During his recent keynote address at Google’s developer conference, company CEO Sundar Pichai said some version of “AI” so many times that his performance was turned into an instantly viral video that clipped together his dozens of references to the technology.
With AI companies one of the few slices of the tech sector still attracting venture capital in a slowing economy, today’s start-ups are quick to claim that they too are incorporating generative AI into their offerings. At last month’s RSA conference, investors in attendance were deluged by pitches from firms claiming to put AI to work in cybersecurity contexts, but all too often, the generative AI tie-ins appeared to be mere hot air.
“What we saw at the show was a lot of people that were slapping a front end on ChatGPT and saying, ‘Hey, look at this cool product,’” said William Kilmer, a cybersecurity-focused investor at the venture capital firm Gallos, to describe the scores of pitches he sat through at RSA with thin claims of using generative AI.
And as companies rush to attract capital and clients, the reality of generative AI can easily be glossed over in marketing copy. “The biggest problem we have here is one of marketing, feeding marketing, feeding marketing,” Guerrero-Sade from SentinelLabs argues. “At this point, people are ready to pack it up, and say the security problem is solved — let’s go! And none of that is remotely true.”
Separating hype from reality, then, represents a tough challenge for investors, technologists, customers and policymakers.
Anne Neuberger, the top cybersecurity adviser at the White House, sees generative AI as a chance to make major improvements in defending computer systems but argues that the technology hasn’t yet delivered to its full potential.
As Neuberger sees it, generative AI could conceivably be used to clean up old code bases, identify vulnerabilities in open-source repositories that lack dedicated maintainers, and even be used to produce provably secure code in formal languages that are hard for people to write. Companies that run extensive end-point security systems — and have access to the data they generate — are in a good position to train effective security models, she believes.
“Bottom line, there’s a lot of opportunity to accelerate cybersecurity and cyberdefense,” Neuberger told CyberScoop. “What we want to do is make sure that in the chase between offense and defense that defense is moving far more quickly. This is especially the case since large language models can be used to generate malware more quickly than before.”
But on the flip side, effectively implementing large language models in security-sensitive contexts faces major challenges. During her time as an official at the National Security Agency, Neuberger said she witnessed these hurdles first-hand when the agency began using language models to supplement the work of analysts, to do language translation and to prioritize what intelligence human analysts should be examining.
Cleaning data to get it usable for machine learning required time and resources, and once the agency rolled out the models for analysts to use some were resistant and were concerned that they could be displaced. “It took a while until it was accepted that such models could triage and to give them a more effective role,” Neuberger said.
For cybersecurity practitioners such as Guerrero-Saade and others who spoke with CyberScoop, some of the most exciting applications for generative AI lie in reverse engineering, the process to understand what a piece of software is trying to do. The malware research community has quickly embraced the use of generative AI, and within a month of ChatGPT’s release a plug-in was released integrating the chatbot with IDA Pro, the software disassembler tool. Even after years of reverse engineering experience, Guerrero-Saade is learning from these tools, such as when he attended a recent training, didn’t understand everything and leaned on ChatGPT to get him started.
ChatGPT really shines when it functions as a kind of “glue logic,” in which it functions as a translator between programs that aren’t associated with one another or a program with a human, says Hammond Pearce, a research assistant professor at New York University’s Center for Cybersecurity. “It’s not that ChatGPT by itself isn’t amazing, because it is, but it’s the combination of ChatGPT with other technologies … that are really going to wow people when new products start coming out.”
For now, defensive cybersecurity applications of generative AI are fairly nascent. Perhaps the most prominent such product — Microsoft’s Security Copilot — remains in private preview with a small number of the company’s clients as it solicits feedback. Using it requires being integrated into the Microsoft security stack and running the company’s other security tools, such as Intune, Defender and Sentinel.
Copilot offers an input system similar to ChatGPT and lets users query a large language model that uses both OpenAI’s GPT-4 and a Microsoft model about security alerts, incidents and malicious code. The goal is to save analysts time by giving them a tool that quickly explains the code or incidents they’re examining and is capable of quickly spitting out analytical products — including close-to-final slide decks.
Chang Kawaguchi, a Microsoft VP and the company’s AI security architect, sees the ability of the current generation of machine learning to work with human language — even with highly technical topics like security — as a “step function change.” The most consistent piece of feedback Kawaguchi’s colleagues have received when they demo Copilot is, “Oh, God, thank you for generating the PowerPoints for us. Like, I hate that part of my job.”
“We couldn’t have done that with last generation’s machine learning,” Kawaguchi told CyberScoop.
Despite their smarts, today’s machine learning tools still have a remarkable penchant for stupidity. Even in Microsoft’s YouTube demo for Copilot, the company’s pitchwoman is at pains to emphasize its limitations and points out that the model refers to Windows 9 — a piece of software that doesn’t exist — as an example of how it can convey false information.
As they are more widely deployed, security experts worry generative AI tools may introduce new, difficult to understand security vulnerabilities. “No one should be trusting these large language models to be reliable right now,” says Jessica Newman, the director of the AI Security Initiative at the University of California at Berkeley.
Newman likens large language models to “instruction following systems” — and that means they can be given instructions to engage in malicious behavior. This category of attack — known as “prompt injection” — leaves models open to manipulation in ways that are difficult to predict. Moreover, AI systems also have typical security vulnerabilities and are susceptible to data poisoning attacks or attacks on their underlying algorithms, Newman points out.
Addressing these vulnerabilities is especially difficult because the nature of large language models means that we often don’t know why they output a given answer — the so-called “black box” problem. Just like a black box, we can’t see inside a large language model and that makes their work difficult to understand. While language models are rapidly advancing, tools to improve their explainability are not moving ahead at the same speed.
“The people who make these systems cannot tell you reliably how they’re making a decision,” Newman said. “That black box nature of these advanced AI systems is kind of unprecedented when dealing with a transformative technology.”
That makes operators of safety critical systems — in, for example, the energy industry — deeply worried about the speed with which large language models are being deployed. “We are concerned by the speed of adoption and the deployment in the field of LLM in the cyber-physical world,” said Leo Simonovich, the vice president and global head for industrial cyber and digital security at Siemens Energy.
AI adoption in the operational technology space — the computers that run critical machinery and infrastructure — has been slow “and rightly so,” Simonovichn said. “In our world, we’ve seen a real hesitancy of AI for security purposes, especially bringing IT security applications that are AI powered into the OT space.”
And as language models deploy more widely, security professionals are also concerned that they lack the right language to describe their work. When LLMs confidently output incorrect information, researchers have taken to describing such statements as “hallucination” — a term that anthropomorphizes a computer system that’s far from human.
These features of LLMs can result in uncanny interactions between man and machine. Kelley, who is the author of the book Practical Cybersecurity Architecture, has asked various LLMs whether she has written a book. Instead of describing the book she did write, LLMs will instead describe a book about zero trust that she hasn’t — but is likely to — write.
“So was that a huge hallucination? Or was that just good old mathematical probability? It was the second but the cool term is hallucination.” Kelley said. “We have to think about how we talk about it.”
Correction, May 23, 2023: An earlier version of this article misspelled William Kilmer’s last name.
The post Reality check: What will generative AI really do for cybersecurity? appeared first on CyberScoop.