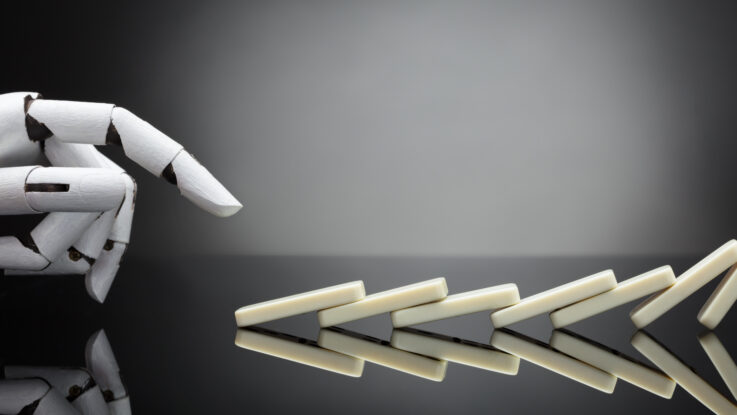
An emerging free tool that analyzes artificial intelligence (AI) models for risk has set a path to become a mainstream part of cybersecurity teams’ toolboxes to tackle AI supply chain risks. Created last March by the AI risk experts at Robust Intelligence, the AI Risk Database has been enhanced with new features and opensourced on GitHub today, in conjunction with new partnership agreements with MITRE and Indiana University that will have the organizations working together to enhance the database’s ability to feed automated AI assessment tools.
“We want this to be VirusTotal for AI,” says Hyrum Anderson, distinguished ML engineer at Robust Intelligence and co-creator of the database.
The database is meant to help the security community discover and report information about security vulnerabilities lurking in public machine learning (ML) models, he says. The database also tracks other factors in these models that threaten reliability and resilience of AI systems, including issues that can cause brittleness, ethical problems, and AI bias.
As Anderson explains, the tool is under development to deal with what is shaping up to be a looming supply chain problem in the world of AI systems. As with many other parts of the software supply chain, AI systems depend on a host of open source components to run their code. But added into that mix is the additional complexity of dependencies on open source ML models and open source data sets used to train data.
“Everyone is reusing models,” Anderson says.
The reuse of models has done a lot to speed up collaborative innovation, but it means that the impact of a flaw in a single model can ripple and reverberate across a wide swath of AI systems.
“AI supply chain security is going to be a huge issue for code, models, and data,” Anderson says.
As a part of today’s release, the AI Risk Database is incorporating a new dependency graph feature created by researchers at the Indiana University Kelley School of Business Data Science and Artificial Intelligence Lab (DSAIL). The feature will make it possible to scan GitHub repositories used to create models to find publicly reported flaws that exist upstream of the delivered model artifact.
Meantime, the partnership with MITRE will bolster the vulnerability research, classification, and risk scoring that powers the AI Risk Database by more closely tying it to the MITRE ATLAS framework. The database is also set to be hosted under the broader set of open source MITRE ATLAS (Adversarial Threat Landscape for Artificial-Intelligence Systems) tools. MITRE is leading the charge in identifying threats and risks to AI with ATLAS, a framework and knowledge base that includes a list of adversary tactics and techniques based on real-world attack observations and AI red teaming.
“This collaboration and release of the AI Risk Database can directly enable more organizations to see for themselves how they are directly at risk and vulnerable in deploying specific types of AI-enabled systems,” said Douglas Robbins, MITRE vice president, engineering and prototyping, in a statement. “As the latest open source tool under MITRE ATLAS, this capability will continue to inform risk assessment and mitigation priorities for organizations around the globe.”
As a part of the announcement, the collaborative team from Robust Intelligence, MITRE, and Indiana University will demo the newly enhanced AI Risk Database at Black Hat Arsenal this week. Anderson will be joined by Christina Liaghati, lead for MITRE ATLAS and the AI strategy execution and operations manager for MITRE’s AI and Autonomy Innovation Center, as well as Sagar Samtani, director of Kelley’s DSAIL at Indiana University, to demonstrate what the database can do during sessions today and tomorrow at Black Hat USA.