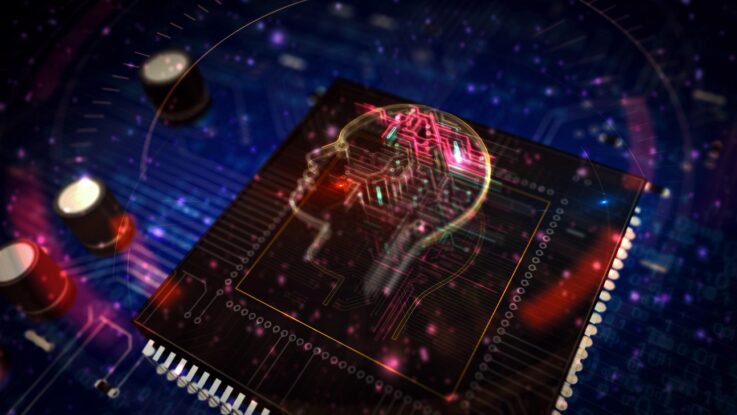
Everyone’s experienced the regret of telling a secret they should’ve kept. Once that information is shared, it can’t be taken back. It’s just part of the human experience.
Now it’s part of the AI experience, too. Whenever someone shares something with a generative AI tool — whether it’s a transcript they’re trying to turn into a paper or financial data they’re attempting to analyze — it cannot be taken back.
Generative AI solutions such as ChatGPT and Google’s Bard have been dominating headlines. The technologies show massive promise for a myriad of use cases and have already begun to change the way we work. But along with these big new opportunities come big risks.
The potential dangers of AI have been discussed at length — probably as much as the technology itself. What will an eventual artificial general intelligence (AGI) mean for humanity? And how will we account for things like the AI alignment problem, which states that, as AI systems become more powerful, they may not do what humans want them to do?
Safety Alignment Concerns
Prior to AI, whenever humans have developed a new technology or product, accompanying safety measures were put into place. Take cars, for example. The earliest versions didn’t feature seatbelts and people were hurt in accidents, which led to seatbelts becoming standard and eventually enforced by law.
Applying safety measures to AI is much more complicated because we’re developing an intangible intelligent entity — there are many unknowns and gray areas. AI has the potential to become a “runaway train” if we’re not careful, and there’s only so much we can do to mitigate its risks.
There’s no telling how the proliferation of generative AI will play out in the coming months and years, but there are a few things companies need to keep in mind as they adopt and experiment with the technology.
Be Careful What You Share and What You Share It With
Organizations must be discerning about which data they share with generative AI models. Many employees are stretched thin, and it can be tempting to reduce one’s workload by offloading tasks to generative AI. But any data shared with these models can be misused or compromised if it falls into the wrong hands. Things such as sensitive (e.g., financial) data, trade secrets, and other confidential business information need to be protected.
One way to reduce this risk is by using private generative AI models. But the problem with this strategy is that — as of now — private models are lacking the easy-to-use user interface (UI) that makes platforms like ChatGPT so popular and appealing. The UI of private models will no doubt improve as companies continue to develop them, but for now, businesses need to have policies in place that prohibit — or at least put parameters around — the use of public models for corporate data.
Be Flexible With AI Policies
AI is a necessity for organizations to stay competitive. This will become more critical as companies attempt to automate more processes, reduce costs, and condense their workforces. As such, organizations need to enact policies around the safe use of AI while simultaneously supporting innovation. There has to be a balance because, if for example, large corporations put too strict of parameters around AI, startups with less regulation could surpass them.
Enforcement of these policies is going to be tricky. As mentioned, private models are cumbersome to use. Everyone wants to get more done faster, so it’ll be tempting to revert to public models like ChatGPT. Companies need to constantly fine-tune their AI best practices, communicate policy changes to employees, and keep an eye out for new private instances that let workers benefit from AI and also keep corporate data secure.
Remember That AI Lies and Lacks Accountability
Despite the promise of AI, it’s not a silver bullet and it is far from perfect. Two particular risks companies need to be aware of are that AI can “hallucinate,” and it’s also nearly impossible to hold people accountable for their use of AI. There’s no way to reliably know who has shared what with any given model. Once data has been shared, you can’t ask the model: How do you know this information? What’s done is done and there’s very little to no accountability.
Additionally, AI can hallucinate if it misinterprets training data. This can result in seemingly authoritative and accurate responses that are essentially bogus. For example, linguist and lexicographer Ben Zimmer asked Google’s Bard about the origin of a fictitious phrase, “argumentative diphthongization.” Despite being entirely made up, Bard produced five paragraphs explaining phony origins of the imaginary phrase. AI has the potential to mislead users with incorrect information, and companies need to stay vigilant because it could have business implications.
AI has quickly become an indispensable business tool and we can soon expect to see significant developments in generative models. Companies need to continue educating themselves and their employees on the benefits and the risks of this technology. By being cautious about information-sharing, staying flexible when it comes to policymaking, and being aware of AI’s limitations, companies can benefit from the perks of AI while minimizing risk.